The Computational Paralinguistics Challenge (ComParE) was organized as part of the ACM Multimedia 2023 conference. The annual challenge deals with states and traits of individuals as manifested in their speech and further signals’ properties. Each year, the organizers introduce tasks, along with data, for the participants to develop solutions.
Our team, consisting of PhD students: Dejan Porjazovski, and Yaroslav Getman, as well as the Research Fellow Tamás Grósz and Professor Mikko Kurimo, participated in the two challenges provided by the organizers:
Requests and Emotion Share.
The Requests sub-challenge involves real interactions in French between call centre agents and customers calling to resolve an issue. The task is further divided into two sub-tasks: Determine whether the customer call concerns a complaint or not, and whether the call concerns membership issues or a process, such as affiliation.
The multilingual Emotion Share task, involving speakers from the USA, South Africa, and Venezuela, tackles a regression problem of recognising the intensity of 9 emotions present in the dataset. The intensities of the emotions that need to be recognized are anger, boredom, calmness, concentration, determination, excitement, interest, sadness, and tiredness.
Our team tackled these issues by utilizing the state-of-the-art wav2vec2 model, along with a Bayesian linear layer. The choice for the appropriate wav2vec2 model, along with the best-performing transformer layer, and the Bayesian linear layer, led to our team winning the Emotion Share sub-challenge:
http://www.compare.openaudio.eu/winners/
For more technical details about the work, see our paper:
https://dl.acm.org/doi/10.1145/3581783.3612848
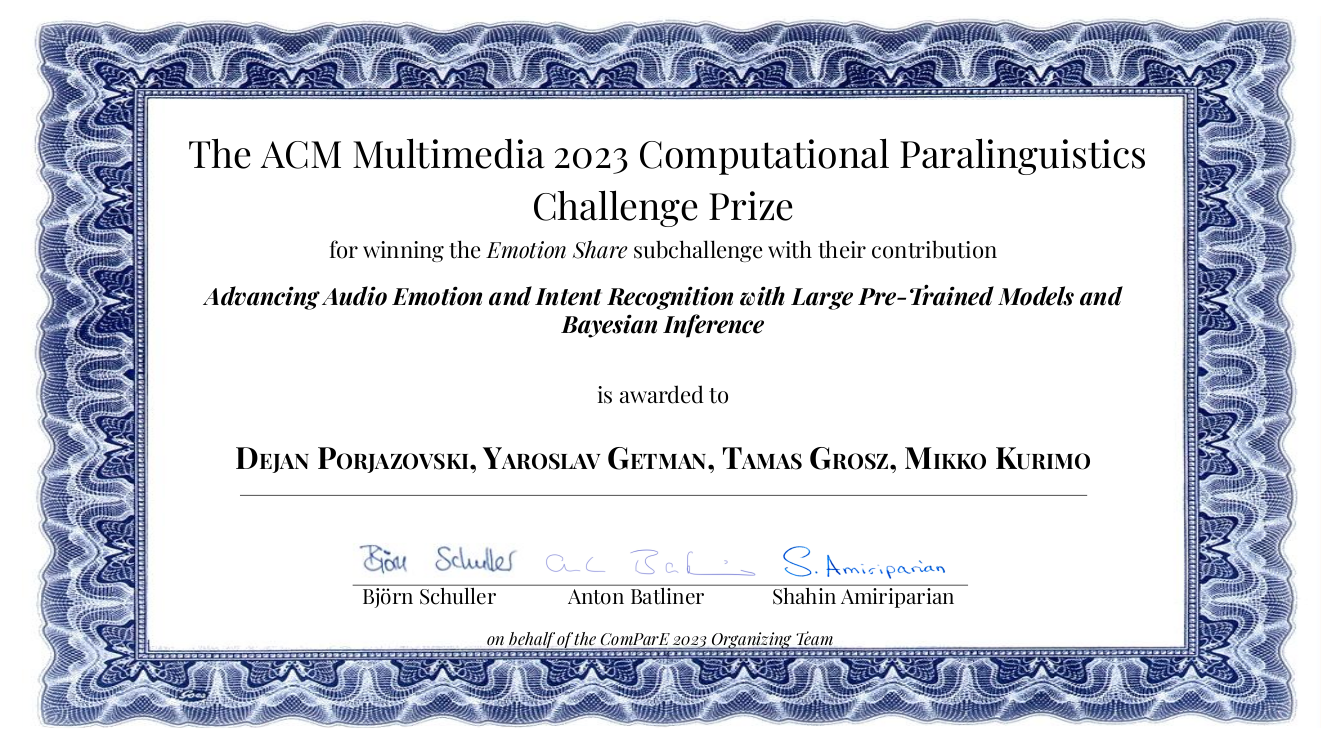
Simultaneously, we also participated in the 4th Multimodal Sentiment Analysis Challenge. The team consisted of PhD students Anja Virkkunen and Dejan Porjazovski, together with Research Fellow Tamás Grósz and Professor Mikko Kurimo.
We chose to tackle two extremely hard problems: Humour and Mimicked Emotions detection using videos. Our solution focused on large, pre-trained models, and we developed a method that could identify relevant parts outputs of these foundation models, making them a bit more transparent. The empirical results demonstrate that these large AIs have smaller specialized outputs that contain relevant information for detecting jokes and emotions of the speakers based on visual and audible cues.
For more details, check out our paper: